Scaling Retail Optimization with Azure AI
Azure automated machine learning (AutoML)
Initially, the company operated its recognition service on virtual machines within Microsoft Azure, leveraging the TensorFlow framework and Mask R-CNN libraries. However, this setup presented limitations in terms of scalability and performance. As the client base utilizing the Image Recognition module expanded and the volume of photo data arrays grew, the company faced prolonged latencies when retrieving recognition results. Additionally, the administrative capabilities of this setup were constrained, and the user interfaces were inconvenient.
The new solution
After a thorough evaluation of various alternatives for a robust Image Recognition (IR) service that offers a seamless end-user experience, the company made the strategic decision to adopt Azure automated machine learning (AutoML). This choice has proven to be highly advantageous, as the new solution powered by Azure AutoML brings several benefits, including cost-saving efficiencies. Notably, it demands less maintenance and offers enhanced control over the testing and training of models.
On the right track
The increase in recognition performance with over 95 percent accuracy and the improved speed of just eight seconds per image processing is indeed impressive. Moreover, beating the goal by 33 percent in terms of processing time is a significant achievement. Field workers can now obtain better results swiftly, which provides retailers and brands with valuable insights into consumer habits. It's essential to continue monitoring the rollout of the Azure AutoML solution and gather feedback from clients to ensure ongoing success.
Proven competence
Awards & certifications
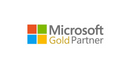
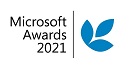